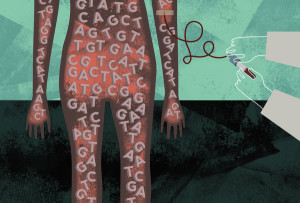
04 May 2016 – In the course of writing my upcoming series “From Barcelona and Zurich: predictive coding, artificial intelligence, and the ‘before-and-after’ ecosystem syndrome”, I revisited my “Rome notes”, so-called because I was in Rome earlier this year at a genetics symposium where scientists from Trinity College Dublin explained the cutting-edge genome technology they used to sequence an ancient British “gladiator’s” genome from Roman York. I have gladiator in quotes because the archaeologists speculated that the skeletons belonged to gladiators, although they could also have been soldiers or criminals. More intriguing was the fact that there was a Middle Eastern body alongside the native British.
As the presenters emphasized, it is the blending of interdisciplinary theories and the technologies of genomics and artificial intelligence and machine learning that is changing medicine, more specifically personal genome services for realizing personal medicine.
My studies have focused on using genomic technology to fully describe cancers and apply that knowledge to guide treatment decisions for individual patients, that from an introduction to “IBM Watson for Oncology” from my colleagues at IBM. And I was fortunate a few years ago to get “interdisciplinary” myself when I was the Project Manager on an e-discovery project involving a dispute over Herceptin which is used to treat patients with metastatic breast cancer. Having worked on several Herceptin cases, I found myself instructing outside counsel on the side effects, interactions and indications of the drug. It was the perfect project for me. I thought I was in heaven.
As I will explain in the series I noted in my first paragraph, the use of artificial intelligence/machine learning in the world of law and e-discovery is interesting but not impressive. As one senior law partner I work with in Europe says “When it becomes push-button, call me”.
Well, at least when one compares its use in science maybe a little primitive. But it will get better. Already artificial intelligence laboratories are succeeding at the test phases of “story telling”: using natural language understanding technology and a form of “early case assessment” technology. Artificial intelligence experts have developed algorithms that can parse structured and unstructured data sets to enable computers to in effect “unite” relationships and “tell a story”. Yes, a very crude use/method of syntactic parsing. And not ready-for-prime-time.
But in medicine … wow. We can now analyse multiple variables from a single specimen, such as changes in DNA, changes in RNA and changes in methylation. Genome-wide scans allow for better systems biology and allow us to learn what’s gone wrong in a particular tumor.
Sequencing tumors is faster, cheaper and easier than ever. With many researchers collecting sequence data and uploading these to public databases such as the The Cancer Genome Atlas, opportunities to describe the many different cancers that arise in breast tissue are upon us. Nicholas Navin, a geneticist at The University of Texas MD Anderson Cancer Center in Houston, Texas, who I follow very closely, was quoted in a recent Nature article:
“The challenge used to be generating the data. Those issues have been resolved. Now the challenge is data processing and data analysing — interpreting the mutations and communicating those to oncologists.”
He noted that University of Pittsburgh researchers are working to link the molecular signatures of people with breast cancer to a host of clinical data, including demographic information associated with risk such as age, ethnicity and body weight. They are mining electronic health records for clinical correlates, treatment interactions and outcomes:
“We’ve got a big haystack and we’re trying to find the needle. But we’re also trying to incriminate the needle, by linking it to lots of things. Collecting all that data from patients’ electronic records adds up. It takes infrastructure — Pittsburgh has already accumulated 5 petabytes, or 5 million gigabytes, which is enough data to overload around 40,000 new iPhone 6 devices.”
Traditionally oncology has been information poor. Cancers have been categorized and treated based on what body part they afflict, how the cells look under a microscope, and how much the tumor had spread, leading to a diagnosis like “Stage 2 colon cancer.” This typology has become more sophisticated over the years, but it still lumps lots of cancers together. Jill Adams, who has written some great pieces for Nature on artificial intelligence technologies and cancer has a great analogy:
“It’s like doing a census of Noah’s ark and concluding that the boat contains a total of one dozen kinds of animals: Ones with feathers and wings, those with six legs and wings, some with fur and four legs, and so on. It’s not wrong, but it gives only a blurry picture of reality.”
In cancer, that has not been good enough. Cancer drugs work (i.e. significantly shrink tumors) a dismal 22 percent of the time, and oncologists have a hard time predicting which one is best for which patient. According to one estimate, $39 billion of the $50 billion spent annually on cancer drugs is wasted in this way. It’s trial-and-error medicine.
DNA sequencing and other biological information technologies are changing that. Already, tumor-gene sequencing reveals that what we have been calling “kidney cancer” or “lung cancer” is, in a sense, a thousand or a million diseases, each with a different pattern of mutations and other molecular mistakes. Each tumor is its own miniature ark, stuffed full of bizarrely dysfunctional cells with a wide range of corrupted DNA. One recent study of kidney cancers found that no two patients had exactly the same set of genetic mistakes; in fact, no two tumors within the same patient had the same mutations. Taking it one step further, one high-resolution DNA-sequencing study of breast cancer last year couldn’t find two cells within one tumor that were genetically identical.
Why does that matter? Jill Adams:
This matters because identifying the mutations often shows how to attack the cancer—where its weaknesses are. Drug developers have now invented dozens of “targeted therapy” drugs that zero in on cells with a particular cancer-related genetic mutation and either kill or disable those cells. Because these drugs are so specific, they are often more effective than older drugs—think of Erbitux for some kinds of colon cancer, or Herceptin for certain types of breast cancer. But in order to use these weapons, you have to first know which enemy you are attacking.
So looking for mutations in breast cancers has become standard, as well as testing some advanced lung cancers for mutations in another gene, EGFR. But these tests only detect one mutation at a time – looking for the keys under the lamppost. A better approach is to do a comprehensive search for mutations.
Granted, using data to change the way we treat cancer will not be as easy as using it to tweak prices of consumer goods on Amazon or avoid traffic jams with a crowdsourced app like Waze. Biology is more complicated than man-made systems. But through comprehensive genetic analysis, due to a phenomenal ability to improve genomic sequencing … we can beat cancer.